1.Kmeans聚类算法简介
kmeans聚类算法是一种迭代求解的聚类分析算法。其实现步骤如下:
(1) 随机选取K个对象作为初始的聚类中心
(2) 计算每个对象与各个种子聚类中心之间的距离,把每个对象分配给距离它最近的聚类中心。
(3) 聚类中心以及分配给它们的对象就代表一个聚类。每分配一个样本,聚类的聚类中心会根据聚类中现有的对象被重新计算。
(4) 重复步骤(2)、(3),直到满足某个终止条件。终止条件可以是聚类中心再发生变化或者误差平方和局部最小等。
2.Kmeans聚类算法的代码实现
(1) 首先,加载需要进行分类的数据集。
data(:,1)=[90,35,52,83,64,24,49,92,99,45,19,38,1,71,56,97,63,... 32,3,34,33,55,75,84,53,15,88,66,41,51,39,78,67,65,25,40,77,... 13,69,29,14,54,87,47,44,58,8,68,81,31];data(:,2)=[33,71,62,34,49,48,46,69,56,59,28,14,55,41,39,... 78,23,99,68,30,87,85,43,88,2,47,50,77,22,76,94,11,80,... 51,6,7,72,36,90,96,44,61,70,60,75,74,63,40,81,4];figure(1)scatter(data(:,1),data(:,2),'LineWidth',2)title("原始数据散点图")
原始数据绘制散点图如下所示:
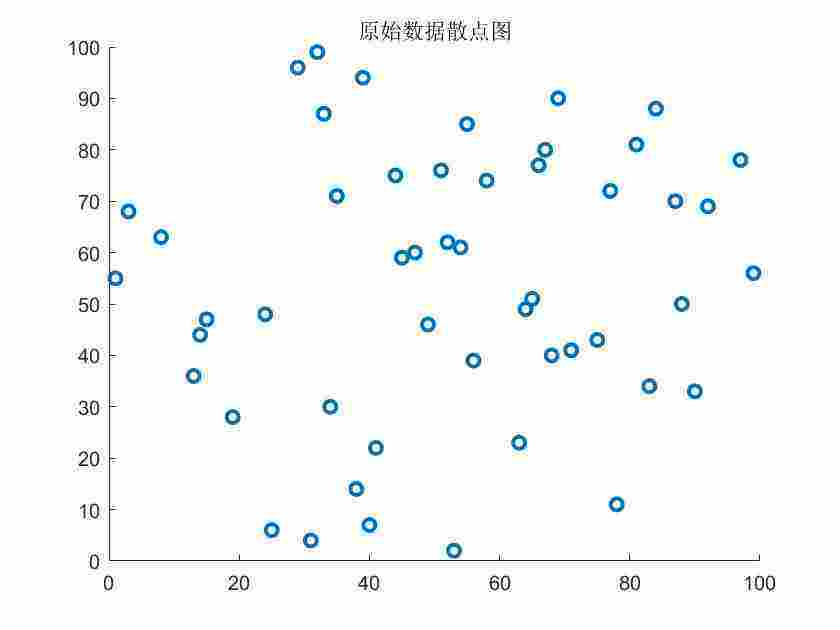
(2) 设置分类数量并调用自己编写的kmeans聚类函数
cluster_num=4;[index_cluster,cluster] = kmeans_func(data,cluster_num);
function [index_cluster,cluster] = kmeans_func(data,cluster_num)%% 原理推导Kmeans聚类算法[m,n]=size(data);cluster=data(randperm(m,cluster_num),:);%从m个点中随机选择cluster_num个点作为初始聚类中心点epoch_max=1000;%最大次数therad_lim=0.001;%中心变化阈值epoch_num=0;while(epoch_num<epoch_max) epoch_num=epoch_num+1; % distance1存储每个点到各聚类中心的欧氏距离 for i=1:cluster_num distance=(data-repmat(cluster(i,:),m,1)).^2; distance1(:,i)=sqrt(sum(distance')); end [~,index_cluster]=min(distance1');%index_cluster取值范围1~cluster_num % cluster_new存储新的聚类中心 for j=1:cluster_num cluster_new(j,:)=mean(data(find(index_cluster==j),:)); end %如果新的聚类中心和上一轮的聚类中心距离和大于therad_lim,更新聚类中心,否则算法结束 if (sqrt(sum((cluster_new-cluster).^2))>therad_lim) cluster=cluster_new; else break; endendend
(3) 对分类结果和最终的聚类中心进行可视化展示
%% 画出聚类效果figure(2)% subplot(2,1,1)a=unique(index_cluster); %找出分类出的个数C=cell(1,length(a));for i=1:length(a) C(1,i)={find(index_cluster==a(i))};endfor j=1:cluster_num data_get=data(C{1,j},:); scatter(data_get(:,1),data_get(:,2),100,'filled','MarkerFaceAlpha',.6,'MarkerEdgeAlpha',.9); hold onend%绘制聚类中心plot(cluster(:,1),cluster(:,2),'ks','LineWidth',2);hold onsc_t=mean(silhouette(data,index_cluster'));title_str=['原理推导K均值聚类',' 聚类数为:',num2str(cluster_num),' SC轮廓系数:',num2str(sc_t)];title(title_str)
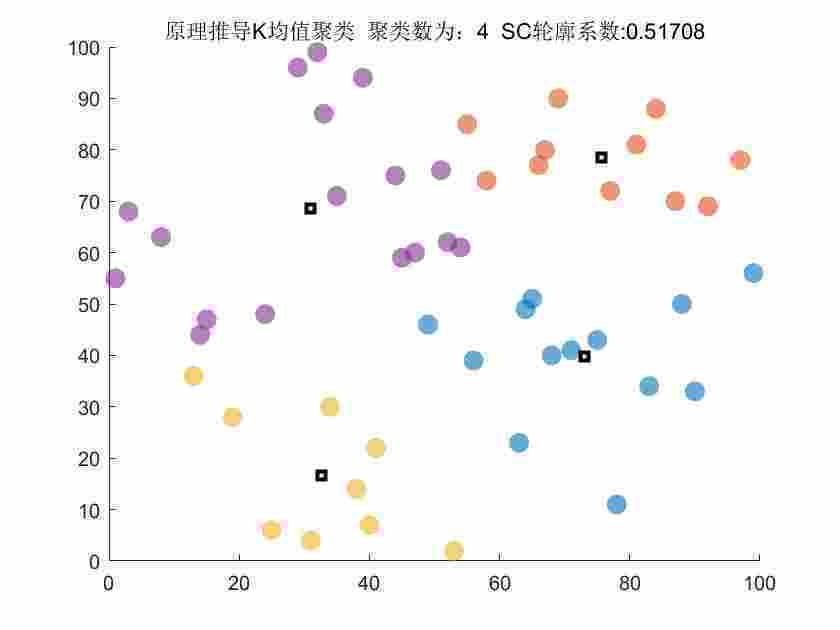
3.完整实现代码
clc;clear;close all;data(:,1)=[90,35,52,83,64,24,49,92,99,45,19,38,1,71,56,97,63,... 32,3,34,33,55,75,84,53,15,88,66,41,51,39,78,67,65,25,40,77,... 13,69,29,14,54,87,47,44,58,8,68,81,31];data(:,2)=[33,71,62,34,49,48,46,69,56,59,28,14,55,41,39,... 78,23,99,68,30,87,85,43,88,2,47,50,77,22,76,94,11,80,... 51,6,7,72,36,90,96,44,61,70,60,75,74,63,40,81,4];figure(1)scatter(data(:,1),data(:,2),'LineWidth',2)title("原始数据散点图")cluster_num=4;[index_cluster,cluster] = kmeans_func(data,cluster_num);%% 画出聚类效果figure(2)% subplot(2,1,1)a=unique(index_cluster); %找出分类出的个数C=cell(1,length(a));for i=1:length(a) C(1,i)={find(index_cluster==a(i))};endfor j=1:cluster_num data_get=data(C{1,j},:); scatter(data_get(:,1),data_get(:,2),100,'filled','MarkerFaceAlpha',.6,'MarkerEdgeAlpha',.9); hold onend%绘制聚类中心plot(cluster(:,1),cluster(:,2),'ks','LineWidth',2);hold onsc_t=mean(silhouette(data,index_cluster'));title_str=['原理推导K均值聚类',' 聚类数为:',num2str(cluster_num),' SC轮廓系数:',num2str(sc_t)];title(title_str)
4. 总结
以上就是matlab实现kmeans聚类算法的全部代码,可在上述代码的基础上进行数据集替换,应用于其它场景。如果有不懂的小伙伴儿,欢迎评论留言或者私信,代码订制也可私信博主。