1.GAM注意力机制:
图像解析:
从整体上可以看出,GAM和CBAM注意力机制还是比较相似的,同样是使用了通道注意力机制和空间注意力机制。但是不同的是对通道注意力和空间注意力的处理。
2.CBAM注意力解析
CBAM = CAM + BAM
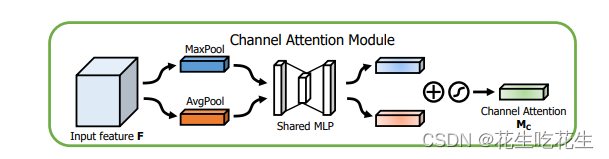
首先对输入特征图进行最大池化和平均池化,再经过MLP分别处理,最终经过Sigmoid激活。对于空间注意力的处理
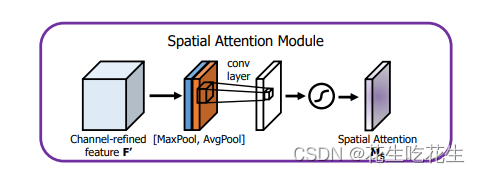
对特征图进行最大池化和平均池化后叠加在一起,再进行卷积,经过Sigmoid激活函数处理。
3.GAM改进
了解了CBAM,我们来看GAM是怎么处理CAM 和SAM的,同样是先通道后空间。
CAM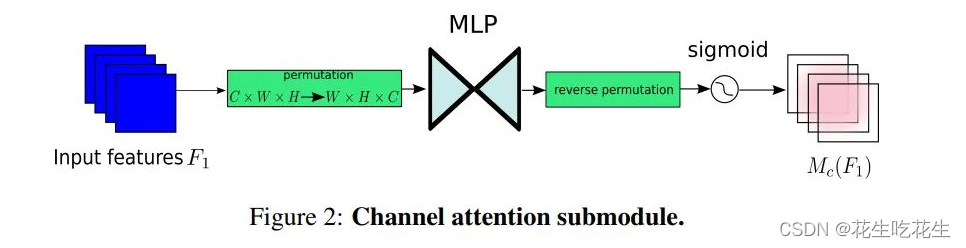
对于输入特征图,首先进行维度转换,经过维度转换的特征图输入到MLP,再转换为原来的维度,进行Sigmoid处理输出。SAM
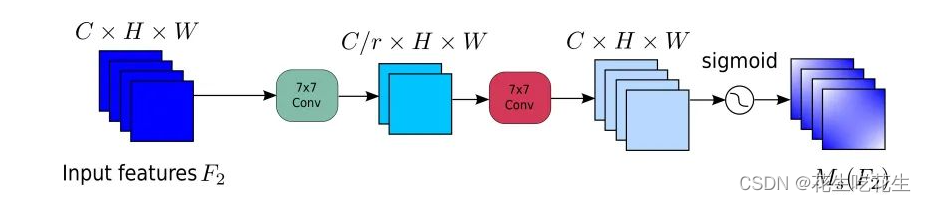
对于SAM,GAM主要使用了卷积处理,对于这里有点像SE注意力机制,先将通道数量减少,再将通道数量增加。首先通过卷积核为7的卷积缩减通道数量,缩小计算量,在经过一个卷积核为7的卷积操作,增加通道数量,保持通道数量的一致。最后经过Sigmoid输出。
4.GAM的pytorch实现
这里给出GAM的pytorch实现代码:代码可能跟官方有些差异,是看图复现的
"""GAM 注意力机制:对CBAM注意力进行改进先通道注意力,再空间注意力"""import torchimport torch.nn as nn# 通道注意力class Channel_Attention(nn.Module): def __init__(self, in_channel, out_channel, ratio=4): super(Channel_Attention, self).__init__() self.fc1 = nn.Linear(in_channel, in_channel // ratio) self.relu = nn.ReLU() self.fc2 = nn.Linear(in_channel // ratio, in_channel) self.sig = nn.Sigmoid() def forward(self, x): # b, c, h, w = x.size() input = x.permute(0, 3, 2, 1) output = self.fc2(self.relu(self.fc1(input))) output = output.permute(0, 3, 2, 1) return output * x# 空间注意力class Spatial(nn.Module): def __init__(self, in_channel, out_channel, ratio, kernel_size=7): super(Spatial, self).__init__() padding = kernel_size // 2 self.conv1 = nn.Conv2d( in_channel, in_channel // ratio, kernel_size=7, padding=padding ) self.bn = nn.BatchNorm2d(in_channel // ratio) self.act = nn.ReLU() self.conv2 = nn.Conv2d( in_channel // ratio, in_channel, kernel_size=kernel_size, padding=padding ) self.bn1 = nn.BatchNorm2d(in_channel) self.sig = nn.Sigmoid() def forward(self, x): conv1 = self.act(self.bn(self.conv1(x))) conv2 = self.bn1(self.conv2(conv1)) output = self.sig(conv2) return x * outputclass GAM(nn.Module): def __init__(self,in_channel, out_channel, ratio = 4, kernel_size = 7): super(GAM, self).__init__() self.channel_attention = Channel_Attention(in_channel,out_channel,ratio) self.spatial_attention = Spatial(in_channel,out_channel,ratio,kernel_size) def forward(self, x): input = self.channel_attention(x) output= self.spatial_attention(input) return outputinput = torch.randn(1, 4, 24, 24).cuda()model = GAM(4, 4).cuda()output = model(input)print(output)print(output.size())# 20220928
5 Reference
YOLOv5使用GAM