图像分类?
文章目录
图像分类? 前言?一、ILSVRC竞赛二、卷积神经网络(CNN)发展1.网络进化2.AlexNet网络3.VGG网络4.GoogLeNet网络5.ResNet网络 总结
前言?
上一章介绍了深度学习的基础内容,这一章来学习一下图像分类的内容。图像分类是计算机视觉中最基础的一个任务,也是几乎所有的基准模型进行比较的任务。从最开始比较简单的10分类的灰度图像手写数字识别任务mnist,到后来更大一点的10分类的 cifar10和100分类的cifar100 任务,到后来的imagenet 任务,图像分类模型伴随着数据集的增长,一步一步提升到了今天的水平。现在,在imagenet 这样的超过1000万图像,超过2万类的数据集中,计算机的图像分类水准已经超过了人类。
一、ILSVRC竞赛
ILSVRC(ImageNet Large Scale Visual Recognition Challenge)是近年来机器视觉领域最受追捧也是最具权威的学术竞赛之一,代表了图像领域的最高水平。ILSVRC竞赛使得深度学习算法得到大力的发展,AI领域迎来了新一轮的热潮,CNN网络也不断迭代,图像分类的准确度也逐年上升,最终超越人类,完成竞赛的使命,2017年已经停办。
ImageNet数据集是ILSVRC竞赛使用的是数据集,由斯坦福大学李飞飞教授主导,包含了超过1400万张全尺寸的有标记图片。ILSVRC比赛会每年从ImageNet数据集中抽出部分样本,以2012年为例,比赛的训练集包含1281167张图片,验证集包含50000张图片,测试集为100000张图片。
卷积神经网络在特征表示上具有极大的优越性,模型提取的特征随着网络深度的增加越来越抽象,越来越能表现图像主题语义,不确定性越少,识别能力越强。AlexNet 的成功证明了CNN 网络能够提升图像分类的效果,其使用了 8 层的网络结构,获得了 2012 年,ImageNet 数据集上图像分类的冠军,为训练深度卷积神经网络模型提供了参考。2014 年,冠军 GoogleNet 另辟蹊径,从设计网络结构的角度来提升识别效果。其主要贡献是设计了 Inception 模块结构来捕捉不同尺度的特征,通过 1×1 的卷积来进行降维。2014 年另外一个工作是 VGG(亚军),进一步证明了网络的深度在提升模型效果方面的重要性。2015 年,最重要的一篇文章是关于深度残差网络 ResNet ,文章提出了拟合残差网络的方法,能够做到更好地训练更深层的网络。 2017年,SENet是ImageNet(ImageNet收官赛)的冠军模型,和ResNet的出现类似,都在很大程度上减小了之前模型的错误率),并且复杂度低,新增参数和计算量小。
历届冠军做法:
二、卷积神经网络(CNN)发展
1.网络进化
?网络:AlexNet–>VGG–>GoogLeNet–>ResNet
?深度:8–>19–>22–>152
✨VGG结构简洁有效
?️性能竞争网络
GoogLeNet:Inception v1–>v4 Split-transform-merge ResNet:ResNet1024–>ResNeXt 深度、宽度、基数2.AlexNet网络
由于受到计算机性能的影响,虽然LeNet在图像分类中取得了较好的成绩,但是并没有引起很多的关注。 知道2012年,Alex等人提出的AlexNet网络在ImageNet大赛上以远超第二名的成绩夺冠,卷积神经网络乃至深度学习重新引起了广泛的关注。
AlexNet包含8层网络,有5个卷积层和3个全连接层AlexNet第一层中的卷积核shape为11X11,第二层的卷积核形状缩小到5X5,之后全部采用3X3的卷积核所有的池化层窗口大小为3X3,步长为2,最大池化采用Relu激活函数,代替sigmoid,梯度计算更简单,模型更容易训练采用Dropout来控制模型复杂度,防止过拟合采用大量图像增强技术,比如翻转、裁剪和颜色变化,扩大数据集,防止过拟合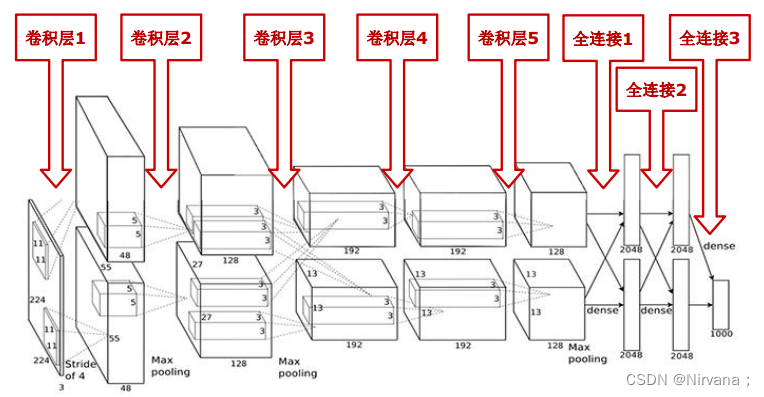
代码实现
# 导入工具包import tensorflow as tffrom tensorflow import kerasfrom tensorflow.keras import layers# 模型构建net = keras.models.Sequential([ # 卷积:卷积核数量96,尺寸11*11,步长4,激活函数relu layers.Conv2D(filters=96, kernel_size=11, strides=4, activation='relu'), # 最大池化:尺寸3*3,步长2 layers.MaxPool2D(pool_size=3, strides=2), # 卷积:卷积核数量256,尺寸5*5,激活函数relu,same卷积 layers.Conv2D(filters=256, kernel_size=5, padding='same', activation='relu'), # 最大池化:尺寸3*3,步长3 layers.MaxPool2D(pool_size=3, strides=2), # 卷积:卷积核数量384,尺寸3,激活函数relu,same卷积 layers.Conv2D(filters=384, kernel_size=3, padding='same', activation='relu'), # 卷积:卷积核数量384,尺寸3,激活函数relu,same卷积 layers.Conv2D(filters=384, kernel_size=3, padding='same', activation='relu'), # 卷积:卷积核数量256,尺寸3,激活函数relu,same卷积 layers.Conv2D(filters=256, kernel_size=3, padding='same', activation='relu'), # 最大池化:尺寸3*3,步长2 layers.MaxPool2D(pool_size=3, strides=2), # 展平特征图 layers.Flatten(), # 全连接:4096神经元,relu layers.Dense(4096, activation='relu'), # 随机失活 layers.Dropout(0.5), layers.Dense(4096, activation='relu'), layers.Dropout(0.5), # 输出层:多分类用softmax,二分类用sigmoid layers.Dense(10, activation='softmax')], name='AlexNet')# 模拟输入x = tf.random.uniform((1, 227, 227, 1))y = net(x)net.summary()
3.VGG网络
VGG网络是在2014年由牛津大学计算机视觉组和谷歌公司的研究员共同开发的。VGG由5层卷积层、3层全连接层、softmax输出层构成,层与层之间使用最大池化分开,所有隐层的激活单元都采用ReLU函数。通过反复堆叠3X3的小卷积核和2X2的最大池化层,VGGNet成功的搭建了16-19层的深度卷积神经网络。VGG的结构图如下:
VGGNet 论文中全部使用了3X3的卷积核和2X2的池化核,通过不断加深网络结构来提升性能。下图所示为 VGGNet 各级别的网络结构图,以及随后的每一级别的参数量,从11层的网络一直到19层的网络都有详尽的性能测试。虽然从A到E每一级网络逐渐变深,但是网络的参数量并没有增长很多,这是因为参数量主要都消耗在最后3个全连接层。前面的卷积部分虽然很深,但是消耗的参数量不大,不过训练比较耗时的部分依然是卷积,因其计算量比较大。这其中的D、E也就是我们常说的 VGGNet-16 和 VGGNet-19。C相比B多了几个1X1的卷积层,1X1卷积的意义主要在于线性变换,而输入通道数和输出通道数不变,没有发生降维。
代码实现 VGG11
#tensorflow基于mnist数据集上的VGG11网络,可以直接运行from tensorflow.examples.tutorials.mnist import input_dataimport tensorflow as tf#tensorflow基于mnist实现VGG11mnist = input_data.read_data_sets('MNIST_data', one_hot=True)x = tf.placeholder(tf.float32, [None, 784])y_ = tf.placeholder(tf.float32, [None, 10])sess = tf.InteractiveSession()#Layer1W_conv1 =tf.Variable(tf.truncated_normal([3, 3, 1, 64],stddev=0.1))b_conv1 = tf.Variable(tf.constant(0.1,shape=[64]))#调整x的大小x_image = tf.reshape(x, [-1,28,28,1])h_conv1 = tf.nn.relu(tf.nn.conv2d(x_image, W_conv1,strides=[1, 1, 1, 1], padding='SAME') + b_conv1)#Layer2 poolingW_conv2 = tf.Variable(tf.truncated_normal([3, 3, 64, 64],stddev=0.1))b_conv2 = tf.Variable(tf.constant(0.1,shape=[64]))h_conv2 = tf.nn.relu(tf.nn.conv2d(h_conv1, W_conv2,strides=[1, 1, 1, 1], padding='SAME') + b_conv2)h_pool2 = tf.nn.max_pool(h_conv2, ksize=[1, 2, 2, 1], strides=[1, 2, 2, 1], padding='SAME')#Layer3W_conv3 = tf.Variable(tf.truncated_normal([3, 3, 64, 128],stddev=0.1))b_conv3 = tf.Variable(tf.constant(0.1,shape=[128]))h_conv3 = tf.nn.relu(tf.nn.conv2d(h_pool2, W_conv3,strides=[1, 1, 1, 1], padding='SAME') + b_conv3)#Layer4 poolingW_conv4 = tf.Variable(tf.truncated_normal([3, 3, 128, 128],stddev=0.1))b_conv4 = tf.Variable(tf.constant(0.1,shape=[128]))h_conv4 = tf.nn.relu(tf.nn.conv2d(h_conv3, W_conv4,strides=[1, 1, 1, 1], padding='SAME') + b_conv4)h_pool4= tf.nn.max_pool(h_conv4, ksize=[1, 2, 2, 1], strides=[1, 2, 2, 1], padding='SAME')#Layer5W_conv5 = tf.Variable(tf.truncated_normal([3, 3, 128, 256],stddev=0.1))b_conv5 = tf.Variable(tf.constant(0.1,shape=[256]))h_conv5 = tf.nn.relu(tf.nn.conv2d(h_pool4, W_conv5,strides=[1, 1, 1, 1], padding='SAME') + b_conv5)#Layer6W_conv6 = tf.Variable(tf.truncated_normal([3, 3, 256, 256],stddev=0.1))b_conv6 = tf.Variable(tf.constant(0.1,shape=[256]))h_conv6 = tf.nn.relu(tf.nn.conv2d(h_conv5, W_conv6,strides=[1, 1, 1, 1], padding='SAME') + b_conv6)#Layer7W_conv7 = tf.Variable(tf.truncated_normal([3, 3, 256, 256],stddev=0.1))b_conv7 = tf.Variable(tf.constant(0.1,shape=[256]))h_conv7 = tf.nn.relu(tf.nn.conv2d(h_conv6, W_conv7,strides=[1, 1, 1, 1], padding='SAME') + b_conv7)#Layer8W_conv8 = tf.Variable(tf.truncated_normal([3, 3, 256, 256],stddev=0.1))b_conv8 = tf.Variable(tf.constant(0.1,shape=[256]))h_conv8 = tf.nn.relu(tf.nn.conv2d(h_conv7, W_conv8,strides=[1, 1, 1, 1], padding='SAME') + b_conv8)h_pool8 = tf.nn.max_pool(h_conv8, ksize=[1, 2, 2, 1], strides=[1, 1, 1, 1], padding='SAME')#Layer9-全连接层W_fc1 = tf.Variable(tf.truncated_normal([7*7*256,1024],stddev=0.1))b_fc1 = tf.Variable(tf.constant(0.1,shape=[1024]))#对h_pool2数据进行铺平h_pool2_flat = tf.reshape(h_pool8, [-1, 7*7*256])#进行relu计算,matmul表示(wx+b)计算h_fc1 = tf.nn.relu(tf.matmul(h_pool2_flat, W_fc1) + b_fc1)keep_prob = tf.placeholder(tf.float32)h_fc1_drop = tf.nn.dropout(h_fc1, keep_prob)#Layer10-全连接层,这里也可以是[1024,其它],大家可以尝试下W_fc2 = tf.Variable(tf.truncated_normal([1024,1024],stddev=0.1))b_fc2 = tf.Variable(tf.constant(0.1,shape=[1024]))h_fc2 = tf.nn.relu(tf.matmul(h_fc1_drop, W_fc2) + b_fc2)h_fc2_drop = tf.nn.dropout(h_fc2, keep_prob)#Layer11-softmax层W_fc3 = tf.Variable(tf.truncated_normal([1024,10],stddev=0.1))b_fc3 = tf.Variable(tf.constant(0.1,shape=[10]))y_conv = tf.matmul(h_fc2_drop, W_fc3) + b_fc3#在这里通过tf.nn.softmax_cross_entropy_with_logits函数可以对y_conv完成softmax计算,同时计算交叉熵损失函数cross_entropy = tf.reduce_mean( tf.nn.softmax_cross_entropy_with_logits(labels=y_, logits=y_conv))#定义训练目标以及加速优化器train_step = tf.train.AdamOptimizer(1e-3).minimize(cross_entropy)#计算准确率correct_prediction = tf.equal(tf.argmax(y_conv,1), tf.argmax(y_,1))accuracy = tf.reduce_mean(tf.cast(correct_prediction, tf.float32))#初始化变量saver = tf.train.Saver()sess.run(tf.global_variables_initializer())for i in range(20000): batch = mnist.train.next_batch(10) if i%100 == 0: train_accuracy = accuracy.eval(feed_dict={ x:batch[0], y_: batch[1], keep_prob: 1.0}) print("step %d, training accuracy %g"%(i, train_accuracy)) train_step.run(feed_dict={x: batch[0], y_: batch[1], keep_prob: 0.5})#保存模型save_path = saver.save(sess, "./model/save_net.ckpt")print("test accuracy %g"%accuracy.eval(feed_dict={ x: mnist.test.images[:3000], y_: mnist.test.labels[:3000], keep_prob: 1.0}))
4.GoogLeNet网络
Google Inception Net通常被称为Google Inception V1,在ILSVRC-2014比赛中由论文<Going deeper with convolutions>提出.
Inception V1有22层,比AlexNet的8层和VGGNet的19层还要深.参数量(500万)仅有AlexNet参数量(6000万)的1/12,但准确率远胜于AlexNet的准确率.
Inception V1降低参数量的目的:
Inception V1网络的特点:
模型层数更深(22层),表达能力更强.去除最后的全连接层,用全局平均池化层(即将图片尺寸变为1X1)来代替它.(借鉴了NIN)使用Inception Module提高了参数利用效率.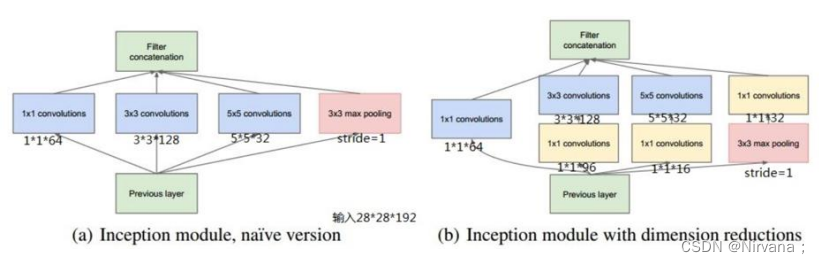
Inception V2网络的特点:Batch Normalization 白化:使每一层的输出都规范化到N(0,1)解决Interal Covariate Shift问题允许较高学习率取代部分Dropout5X5卷积核–>2个3X3卷积核
Inception V3网络的特点:
GoogLeNet网络结构:
对于我们搭建的Inception模块,所需要使用到参数有#1x1, #3x3reduce, #3x3, #5x5reduce, #5x5, poolproj,这6个参数,分别对应着所使用的卷积核个数,参数设置如下表所示:
代码实现 Inception V3
import tensorflow as tfslim = tf.contrib.slimtrunc_normal = lambda stddev: tf.truncated_normal_initializer(0.0, stddev)# 生成默认参数def inception_v3_arg_scope(weight_decay=0.00004, # L2正则weight_decay stddev=0.1, # 标准差 batch_norm_var_collection='moving_vars'): batch_norm_params = { 'decay': 0.9997, 'epsilon':0.001, 'updates_collections': tf.GraphKeys.UPDATE_OPS, 'variables_collections':{ 'beta': None, 'gamma': None, 'moving_mean': [batch_norm_var_collection], 'moving_variance': [batch_norm_var_collection], } } # 提供了新的范围名称scope name # 对slim.conv2d和slim.fully_connected两个函数的参数自动赋值 with slim.arg_scope([slim.conv2d, slim.fully_connected], weights_regularizer=slim.l2_regularizer(weight_decay)): with slim.arg_scope( [slim.conv2d], # 对卷积层的参数赋默认值 weights_initializer=tf.truncated_normal_initializer(stddev=stddev), # 权重初始化器 activation_fn=tf.nn.relu, # 激活函数用ReLU normalizer_params=batch_norm_params) as sc: # 标准化器参数用batch_norm_params return sc# inputs为输入图片数据的tensor(299x299x3),scope为包含了函数默认参数的环境def inception_v3_base(inputs, scope=None): # 保存某些关键节点 end_points = {} # 定义InceptionV3的网络结构 with tf.variable_scope(scope, 'InceptionV3', [inputs]): # 设置卷积/最大池化/平均池化的默认步长为1,padding模式为VALID # 设置Inception模块组的默认参数 with slim.arg_scope([slim.conv2d, # 创建卷积层 slim.max_pool2d, # 输出的通道数 slim.avg_pool2d], # 卷积核尺寸 stride=1, # 步长 padding='VALID'): # padding模式 # 经3个3x3的卷积层后,输入数据(299x299x3)变为(35x35x192),空间尺寸降低,输出通道增加 net = slim.conv2d(inputs, 32, [3,3], stride=2, scope='Conv2d_1a_3x3') net = slim.conv2d(net, 32, [3, 3], scope='Conv2d_2a_3x3') net = slim.conv2d(net, 64, [3, 3], padding='SAME', scope='Conv2d_2b_3x3') net = slim.max_pool2d(net, [3, 3], stride=2, scope='MaxPool_3a_3x3') net = slim.conv2d(net, 80, [1, 1], scope='Conv2d_3b_1x1') net = slim.conv2d(net, 192, [3, 3], scope='Conv2d_4a_3x3') net = slim.max_pool2d(net, [3, 3], stride=2, scope='MaxPool_5a_3x3') # 设置卷积/最大池化/平均池化的默认步长为1,padding模式为SAME # 步长为1,padding模式为SAME,所以图像尺寸不会变,仍为35x35 with slim.arg_scope([slim.conv2d, slim.max_pool2d, slim.avg_pool2d], stride=1, padding='SAME'): # 设置Inception Moduel名称为Mixed_5b with tf.variable_scope('Mixed_5b'): # 第1个分支:64输出通道的1x1卷积 with tf.variable_scope('Branch_0'): branch_0 = slim.conv2d(net, 64, [1, 1], scope='Conv2d_0a_1x1') # 第2个分支:48输出通道的1x1卷积,连接64输出通道的5x5卷积 with tf.variable_scope('Branch_1'): branch_1 = slim.conv2d(net, 48, [1, 1], scope='Con2d_0a_1x1') branch_1 = slim.conv2d(branch_1, 64, [5, 5], scope='Conv2d_0b_5x5') # 第3个分支:64输出通道的1x1卷积,连接两个96输出通道的3x3卷积 with tf.variable_scope('Branch_2'): branch_2 = slim.conv2d(net, 64, [1, 1], scope='Conv2d_0a_1x1') branch_2 = slim.conv2d(branch_2, 96, [3, 3], scope='Conv2d_0b_3x3') branch_2 = slim.conv2d(branch_2, 96, [3, 3], scope='Conv2d_0c_3x3') # 第4个分支:3x3的平均池化,连接32输出通道的1x1卷积 with tf.variable_scope('Branch_3'): branch_3 = slim.avg_pool2d(net, [3, 3], scope='AvgPool_0a_3x3') branch_3 = slim.conv2d(branch_3, 32, [1, 1], scope='Conv2d_0b_1x1') # 4个分支输出通道数之和=64+64+96+32=256,输出tensor为35x35x256 net = tf.concat([branch_0, branch_1, branch_2, branch_3], 3) # 第1个Inception模块组的第2个Inception Module with tf.variable_scope('Mixed_5c'): # 第1个分支:64输出通道的1x1卷积 with tf.variable_scope('Branch_0'): branch_0 = slim.conv2d(net, 64, [1, 1], scope='Conv2d_0a_1x1') # 第2个分支:48输出通道的1x1卷积,连接64输出通道的5x5卷积 with tf.variable_scope('Branch_1'): branch_1 = slim.conv2d(net, 48, [1, 1], scope='Conv2d_0b_1x1') branch_1 = slim.conv2d(branch_1, 64, [5, 5], scope='Conv2d_0c_5x5') # 第3个分支:64输出通道的1x1卷积,连接两个96输出通道的3x3卷积 with tf.variable_scope('Branch_2'): branch_2 = slim.conv2d(net, 64, [1, 1], scope='Conv2d_0a_1x1') branch_2 = slim.conv2d(branch_2, 96, [3, 3], scope='Conv2d_0b_3x3') branch_2 = slim.conv2d(branch_2, 96, [3, 3], scope='Conv2d_0c_3x3') # 第4个分支:3x3的平均池化,连接64输出通道的1x1卷积 with tf.variable_scope('Branch_3'): branch_3 = slim.avg_pool2d(net, [3, 3], scope='AvgPool_0a_3x3') branch_3 = slim.conv2d(branch_3, 64, [1, 1], scope='Conv2d_0b_1x1') # 输出tensor尺寸为35x35x288 net = tf.concat([branch_0, branch_1, branch_2, branch_3], 3) # 第1个Inception模块组的第3个Inception Module with tf.variable_scope('Mixed_5d'): # 第1个分支:64输出通道的1x1卷积 with tf.variable_scope('Branch_0'): branch_0 = slim.conv2d(net, 64, [1, 1], scope='Conv2d_0a_1x1') # 第2个分支:48输出通道的1x1卷积,连接64输出通道的5x5卷积 with tf.variable_scope('Branch_1'): branch_1 = slim.conv2d(net, 48, [1, 1], scope='Conv2d_0a_1x1') branch_1 = slim.conv2d(branch_1, 64, [5, 5], scope='Conv2d_0b_5x5') # 第3个分支:64输出通道的1x1卷积,连接两个96输出通道的3x3卷积 with tf.variable_scope('Branch_2'): branch_2 = slim.conv2d(net, 64, [1, 1], scope='Conv2d_0a_1x1') branch_2 = slim.conv2d(branch_2, 96, [3, 3], scope='Conv2d_0b_3x3') branch_2 = slim.conv2d(branch_2, 96, [3, 3], scope='Conv2d_0c_3x3') # 第4个分支:3x3的平均池化,连接64输出通道的1x1卷积 with tf.variable_scope('Branch_3'): branch_3 = slim.avg_pool2d(net, [3, 3], scope='AvgPool_0a_3x3') branch_3 = slim.conv2d(branch_3, 64, [1, 1], scope='Conv2d_0b_1x1') # 输出tensor尺寸为35x35x288 net = tf.concat([branch_0, branch_1, branch_2, branch_3], 3) # 第2个Inception模块组 with tf.variable_scope('Mixed_6a'): # 第1个分支:3x3卷积,步长为2,padding模式为VALID,因此图像被压缩为17x17 with tf.variable_scope('Branch_0'): branch_0 = slim.conv2d(net, 384, [3, 3], stride=2 , padding='VALID', scope='Conv2d_1a_1x1') # 第2个分支:64输出通道的1x1卷积,连接2个96输出通道的3x3卷积 with tf.variable_scope('Branch_1'): branch_1 = slim.conv2d(net, 64, [1, 1], scope='Conv2d_0a_1x1') branch_1 = slim.conv2d(branch_1, 96, [3, 3], scope='Conv2d_0b_3x3') # 步长为2,padding模式为VALID,因此图像被压缩为17x17 branch_1 = slim.conv2d(branch_1, 96, [3, 3], stride=2, padding='VALID', scope='Conv2d_1a_1x1') # 第3个分支:3x3的最大池化层,步长为2,padding模式为VALID,因此图像被压缩为17x17x256 with tf.variable_scope('Branch_2'): branch_2 = slim.max_pool2d(net, [3, 3], stride=2, padding='VALID', scope='MaxPool_1a_3x3') net = tf.concat([branch_0, branch_1, branch_2], 3) # 第2个Inception模块组,包含5个Inception Module with tf.variable_scope('Mixed_6b'): # 第1个分支:192输出通道的1x1卷积 with tf.variable_scope('Branch_0'): branch_0 = slim.conv2d(net, 192, [1, 1], scope='Conv2d_0a_1x1') # 第2个分支:128输出通道的1x1卷积,接128输出通道的1x7卷积,接192输出通道的7x1卷积 with tf.variable_scope('Branch_1'): branch_1 = slim.conv2d(net, 128, [1, 1], scope='Conv2d_0a_1x1') branch_1 = slim.conv2d(branch_1, 128, [1, 7], scope='Conv2d_0b_1x7') branch_1 = slim.conv2d(branch_1, 192, [7, 1], scope='Conv2d_0c_7x1') with tf.variable_scope('Branch_2'): branch_2 = slim.conv2d(net, 128, [1, 1], scope='Conv2d_0a_1x1') branch_2 = slim.conv2d(branch_2, 128, [7, 1], scope='Conv2d_0b_7x1') branch_2 = slim.conv2d(branch_2, 128, [1, 7], scope='Conv2d_0c_1x7') branch_2 = slim.conv2d(branch_2, 128, [7, 1], scope='Conv2d_0d_7x1') branch_2 = slim.conv2d(branch_2, 192, [1, 7], scope='Conv2d_0e_1x7') with tf.variable_scope('Branch_3'): branch_3 = slim.avg_pool2d(net, [3, 3], scope='AvgPool_0a_3x3') branch_3 = slim.conv2d(branch_3, 192, [1, 1], scope='Conv2d_0b_1x1') # 输出tensor尺寸=17x17x(192+192+192+192)=17x17x768 net = tf.concat([branch_0, branch_1, branch_2, branch_3], 3) # 经过一个Inception Module输出tensor尺寸不变,但特征相当于被精炼类一遍 # 第3个Inception模块组 with tf.variable_scope('Mixed_6c'): with tf.variable_scope('Branch_0'): branch_0 = slim.conv2d(net, 192, [1, 1], scope='Conv2d_0a_1x1') with tf.variable_scope('Branch_1'): branch_1 = slim.conv2d(net, 160, [1, 1], scope='Conv2d_0a_1x1') branch_1 = slim.conv2d(branch_1, 160, [1, 7], scope='Conv2d_0b_1x7') branch_1 = slim.conv2d(branch_1, 192, [7, 1], scope='Conv2d_0c_7x1') with tf.variable_scope('Branch_2'): branch_2 = slim.conv2d(net, 160, [1, 1], scope='Conv2d_0a_1x1') branch_2 = slim.conv2d(branch_2, 160, [7, 1], scope='Conv2d_0b_7x1') branch_2 = slim.conv2d(branch_2, 160, [1, 7], scope='Conv2d_0c_1x7') branch_2 = slim.conv2d(branch_2, 160, [7, 1], scope='Conv2d_0d_7x1') branch_2 = slim.conv2d(branch_2, 192, [1, 7], scope='Conv2d_0e_1x7') with tf.variable_scope('Branch_3'): branch_3 = slim.avg_pool2d(net, [3, 3], scope='AvgPool_0a_3x3') branch_3 = slim.conv2d(branch_3, 192, [1, 1], scope='Conv2d_0b_1x1') # 输出tensor尺寸为17x17x768 net = tf.concat([branch_0, branch_1, branch_2, branch_3], 3) # 第4个Inception模块组 with tf.variable_scope('Mixed_6d'): with tf.variable_scope('Branch_0'): branch_0 = slim.conv2d(net, 192, [1, 1], scope='Conv2d_0a_1x1') with tf.variable_scope('Branch_1'): branch_1 = slim.conv2d(net, 160, [1, 1], scope='Conv2d_0a_1x1') branch_1 = slim.conv2d(branch_1, 160, [1, 7], scope='Conv2d_0b_1x7') branch_1 = slim.conv2d(branch_1, 192, [7, 1], scope='Conv2d_0c_7x1') with tf.variable_scope('Branch_2'): branch_2 = slim.conv2d(net, 160, [1, 1], scope='Conv2d_0a_1x1') branch_2 = slim.conv2d(branch_2, 160, [7, 1], scope='Conv2d_0b_7x1') branch_2 = slim.conv2d(branch_2, 160, [1, 7], scope='Conv2d_0c_1x7') branch_2 = slim.conv2d(branch_2, 160, [7, 1], scope='Conv2d_0d_7x1') branch_2 = slim.conv2d(branch_2, 192, [1, 7], scope='Conv2d_0e_1x7') with tf.variable_scope('Branch_3'): branch_3 = slim.avg_pool2d(net, [3, 3], scope='AvgPool_0a_3x3') branch_3 = slim.conv2d(branch_3, 192, [1, 1], scope='Conv2d_0b_1x1') # 输出tensor尺寸为17x17x768 net = tf.concat([branch_0, branch_1, branch_2, branch_3], 3) # 第5个Inception模块组 with tf.variable_scope('Mixed_6e'): with tf.variable_scope('Branch_0'): branch_0 = slim.conv2d(net, 192, [1, 1], scope='Conv2d_0a_1x1') with tf.variable_scope('Branch_1'): branch_1 = slim.conv2d(net, 192, [1, 1], scope='Conv2d_0a_1x1') branch_1 = slim.conv2d(branch_1, 192, [1, 7], scope='Conv2d_0b_1x7') branch_1 = slim.conv2d(branch_1, 192, [7, 1], scope='Conv2d_0c_7x1') with tf.variable_scope('Branch_2'): branch_2 = slim.conv2d(net, 192, [1, 1], scope='Conv2d_0a_1x1') branch_2 = slim.conv2d(branch_2, 192, [7, 1], scope='Conv2d_0b_7x1') branch_2 = slim.conv2d(branch_2, 192, [1, 7], scope='Conv2d_0c_1x7') branch_2 = slim.conv2d(branch_2, 192, [7, 1], scope='Conv2d_0d_7x1') branch_2 = slim.conv2d(branch_2, 192, [1, 7], scope='Conv2d_0e_1x7') with tf.variable_scope('Branch_3'): branch_3 = slim.avg_pool2d(net, [3, 3], scope='AvgPool_0a_3x3') branch_3 = slim.conv2d(branch_3, 192, [1, 1], scope='Conv2d_0b_1x1') # 输出tensor尺寸为17x17x768 net = tf.concat([branch_0, branch_1, branch_2, branch_3], 3) # 将Mixed_6e存储于end_points中 end_points['Mixed_6e'] = net # 第3个Inception模块 # 第1个Inception模块组 with tf.variable_scope('Mixed_7a'): # 第1个分支:192输出通道的1x1卷积,接320输出通道的3x3卷积 步长为2 with tf.variable_scope('Branch_0'): branch_0 = slim.conv2d(net, 192, [1, 1], scope='Conv2d_0a_1x1') branch_0 = slim.conv2d(branch_0, 320, [3, 3], stride=2, padding='VALID', scope='Conv2d_0a_3x3') # 第2个分支:4个卷积层 with tf.variable_scope('Branch_1'): # 192输出通道的1x1卷积 branch_1 = slim.conv2d(net, 192, [1, 1], scope='Conv2d_0a_1x1') # 192输出通道的1x7卷积 branch_1 = slim.conv2d(branch_1, 192, [1, 7], scope='Conv2d_0b_1x7') # 192输出通道的7x1卷积 branch_1 = slim.conv2d(branch_1, 192, [7, 1], scope='Conv2d_0c_7x1') # 192输出通道的3x3卷积 步长为2,输出8x8x192 branch_1 = slim.conv2d(branch_1, 192, [3, 3], stride=2, padding='VALID', scope='Conv2d_1a_3x3') # 第3个分支:3x3的最大池化层,输出8x8x768 with tf.variable_scope('Branch_2'): branch_2 = slim.max_pool2d(net, [3, 3], stride=2, padding='VALID', scope='MaxPool_1a_3x3') # 输出tensor尺寸:8x8x(320+192+768)=8x8x1280,尺寸缩小,通道数增加 net = tf.concat([branch_0, branch_1, branch_2], 3) # 第2个Inception模块组 with tf.variable_scope('Mixed_7b'): # 第1个分支:320输出通道的1x1卷积 with tf.variable_scope('Branch_0'): branch_0 = slim.conv2d(net, 320, [1, 1], scope='Conv2d_0a_1x1') # 第2个分支:384输出通道的1x1卷积 # 分支内拆分为两个分支:384输出通道的1x3卷积+384输出通道的3x1卷积 with tf.variable_scope('Branch_1'): branch_1 = slim.conv2d(net, 384, [1, 1], scope='Conv2d_0a_1x1') branch_1 = tf.concat([ slim.conv2d(branch_1, 384, [1, 3], scope='Conv2d_0b_1x3'), slim.conv2d(branch_1, 384, [3, 1], scope='Conv2d_0b_3x1')], 3) # 第3个分支:448输出通道的1x1卷积,接384输出通道的3x3卷积,分支内拆分为两个分支 with tf.variable_scope('Branch_2'): branch_2 = slim.conv2d(net, 448, [1, 1], scope='Conv2d_0a_1x1') branch_2 = slim.conv2d(branch_2, 384, [3, 3], scope='Conv2d_0b_3x3') # 分支内拆分为两个分支:384输出通道的1x3卷积+384输出通道的3x1卷积 branch_2 = tf.concat([ slim.conv2d(branch_2, 384, [1, 3], scope='Conv2d_0c_1x3'), slim.conv2d(branch_2, 384, [3, 1], scope='Conv2d_0d_3x1')], 3) # 第4个分支:3x3的平均池化层,接192输出通道的1x1卷积,输出8x8x768 with tf.variable_scope('Branch_3'): branch_3 = slim.avg_pool2d(net, [3, 3], scope='AvgPool_0a_3x3') branch_3 = slim.conv2d(branch_3, 192, [1, 1], scope='Conv2d_0b_1x1') # 输出tensor尺寸:8x8x(320+768+768+192)=8x8x2048 net = tf.concat([branch_0, branch_1, branch_2, branch_3], 3) # 第3个Inception模块组 with tf.variable_scope('Mixed_7c'): # 第1个分支:320输出通道的1x1卷积 with tf.variable_scope('Branch_0'): branch_0 = slim.conv2d(net, 320, [1, 1], scope='Conv2d_0a_1x1') # 第2个分支:384输出通道的1x1卷积 # 分支内拆分为两个分支:384输出通道的1x3卷积+384输出通道的3x1卷积 with tf.variable_scope('Branch_1'): branch_1 = slim.conv2d(net, 384, [1, 1], scope='Conv2d_0a_1x1') branch_1 = tf.concat([ slim.conv2d(branch_1, 384, [1, 3], scope='Conv2d_0b_1x3'), slim.conv2d(branch_1, 384, [3, 1], scope='Conv2d_0c_3x1')], 3) # 第3个分支:448输出通道的1x1卷积,接384输出通道的3x3卷积,分支内拆分为两个分支 with tf.variable_scope('Branch_2'): branch_2 = slim.conv2d(net, 448, [1, 1], scope='Conv2d_0a_1x1') branch_2 = slim.conv2d(branch_2, 384, [3, 3], scope='Conv2d_0b_3x3') # 分支内拆分为两个分支:384输出通道的1x3卷积+384输出通道的3x1卷积 branch_2 = tf.concat([ slim.conv2d(branch_2, 384, [1, 3], scope='Conv2d_0c_1x3'), slim.conv2d(branch_2, 384, [3, 1], scope='Conv2d_0d_3x1')], 3) # 第4个分支:3x3的平均池化层,接192输出通道的1x1卷积,输出8x8x768 with tf.variable_scope('Branch_3'): branch_3 = slim.avg_pool2d(net, [3, 3], scope='AvgPool_0a_3x3') branch_3 = slim.conv2d(branch_3, 192, [1, 1], scope='Conv2d_0b_1x1') # 输出tensor尺寸:8x8x(320+768+768+192)=8x8x2048 net = tf.concat([branch_0, branch_1, branch_2, branch_3], 3) return net, end_points# 全局平均池化def inception_v3(inputs, num_classes=1000, # 最后分类数量 is_training=True, # 是否是训练过程的标志 dropout_keep_prob=0.8, # Dropout保留节点的比例 prediction_fn=slim.softmax,# 进行分类的函数 spatial_squeeze=True, # 是否对输出进行squeeze操作,即去除维数为1的维度 reuse=None, # tf.variable_scope的reuse默认值 scope='InceptionV3'): # tf.variable_scope的scope默认值 with tf.variable_scope(scope, 'InceptionV3', [inputs, num_classes], reuse=reuse) as scope: with slim.arg_scope([slim.batch_norm, slim.dropout], is_training=is_training): net, end_points = inception_v3_base(inputs, scope=scope) # 设置卷积/最大池化/平均池化的默认步长为1,padding模式为SAME with slim.arg_scope([slim.conv2d, slim.max_pool2d, slim.avg_pool2d], stride=1, padding='SAME'): aux_logits = end_points['Mixed_6e'] # 辅助分类节点 with tf.variable_scope('AuxLogits'): # 5x5的平均池化,步长设为3,padding模式设为VALID aux_logits = slim.avg_pool2d(aux_logits, [5, 5], stride=3, padding='VALID', scope='AvgPool_1a_5x5') aux_logits = slim.conv2d(aux_logits, 128, [1, 1], scope='Conv2d_1b_1x1') aux_logits = slim.conv2d(aux_logits,768, [5, 5], weights_initializer=trunc_normal(0.01), padding='VALID', scope='Conv2d_2a_5x5') aux_logits = slim.conv2d(aux_logits, num_classes, [1, 1], activation_fn=None, normalizer_fn=None, weights_initializer=trunc_normal(0.001), scope='Conv2d_2b_1x1') if spatial_squeeze: # 进行squeeze操作,去除维数为1的维度 aux_logits = tf.squeeze(aux_logits, [1, 2], name='SpatialSqueeze') end_points['AuxLogits'] = aux_logits # 处理正常的分类预测 with tf.variable_scope('Logits'): # 8x8的平均池化层 net = slim.avg_pool2d(net, [8, 8], padding='VALID', scope='AvgPool_1a_8x8') # Dropout层 net = slim.dropout(net, keep_prob=dropout_keep_prob, scope='Dropout_1b') end_points['PreLogits'] = net logits = slim.conv2d(net, num_classes, [1, 1], activation_fn= None, normalizer_fn=None,scope='Conv2d_1c_1x1') if spatial_squeeze: # 进行squeeze操作,去除维数为1的维度 logits = tf.squeeze(logits, [1, 2], name='SpatialSqueeze') # 辅助节点 end_points['Logits'] = logits # 利用Softmax对结果进行分类预测 end_points['Predictions'] = prediction_fn(logits, scope='Predictions') return logits, end_pointsimport mathfrom datetime import datetimeimport time# 评估每轮计算占用的时间# 输入TensorFlow的Session,需要测评的算子target,测试的名称info_stringdef time_tensorflow_run(session, target, info_string): # 定义预热轮数(忽略前10轮,不考虑显存加载等因素的影响) num_steps_burn_in = 10 total_duration = 0.0 total_duration_squared = 0.0 for i in range(num_batches + num_steps_burn_in): start_time = time.time() _ = session.run(target) # 持续时间 duration = time.time()- start_time if i >= num_steps_burn_in: # 只考量10轮迭代之后的计算时间 if not i % 10: print '%s: step %d, duration = %.3f' % (datetime.now().strftime('%X'), i - num_steps_burn_in, duration) # 记录总时间 total_duration += duration total_duration_squared += duration * duration # 计算每轮迭代的平均耗时mn,和标准差sd mn = total_duration / num_batches vr = total_duration_squared / num_batches - mn * mn sd = math.sqrt(vr) # 打印出每轮迭代耗时 print '%s: %s across %d steps, %.3f +/- %.3f sec / batch' % (datetime.now().strftime('%X'), info_string, num_batches, mn, sd)# Inception V3运行性能测试if __name__ == '__main__': batch_size = 32 height, width = 299, 299 inputs = tf.random_uniform((batch_size, height, width, 3)) with slim.arg_scope(inception_v3_arg_scope()): # 传入inputs获取logits,end_points logits, end_points = inception_v3(inputs, is_training=False) # 初始化 init = tf.global_variables_initializer() sess = tf.Session() sess.run(init) num_batches = 100 # 测试Inception V3的forward性能 time_tensorflow_run(sess, logits, 'Forward')
5.ResNet网络
ResNet是一个应用十分广泛的卷积神经网络的特征提取网络,在2016年由大名鼎鼎的何恺明(He-Kaiming)及其团队提出,他曾以第一作者身份拿过2次CVPR最佳论文奖(2009年和2016年),其中2016年CVPR最佳论文就是这个深度残差网络。
ResNet残差网络特点:
各种ResNet残差网络:
以Resnet18为例,它是由残差块堆叠而成的网络–1个卷积层+8个残差块(每个残差块有2个卷积层)+1个全连接层,如下图:
代码实现 ResNet18
#coding:utf-8import osos.environ['TF_CPP_MIN_LOG_LEVEL'] = '2'import tensorflow as tffrom tensorflow import kerasfrom tensorflow.keras import layers, Sequential#构建残差块class BasicBlock(layers.Layer): def __init__(self, filter_num, stride=1): super(BasicBlock, self).__init__() #卷积层(过滤器尺寸3*3,过滤器个数filter_num(可变),步长为stride(可变),padding为same(输出尺寸=输入尺寸/步长) self.conv1 = layers.Conv2D(filter_num, (3, 3), strides=stride, padding='same') #BatchNormalization标准化 self.bn1 = layers.BatchNormalization() #激活函数选择relu self.relu = layers.Activation('relu') #卷积层(过滤器尺寸3*3,过滤器个数filter_num(可变),步长为1,padding为same(输出尺寸=输入尺寸/步长) self.conv2 = layers.Conv2D(filter_num, (3, 3), strides=1, padding='same') self.bn2 = layers.BatchNormalization() #对步长进行判断,以减少参数量(如果步长等于1,则为原x;否则将x输入一个过滤器为1、步长为stride的卷积层中) if stride != 1: #建立一个容器 self.downsample = Sequential() # 卷积层(过滤器尺寸1*1,过滤器个数filter_num(可变),步长为stride(可变));输出尺寸=向下取整((输入尺寸-过滤器尺寸)/步长)+1) self.downsample.add(layers.Conv2D(filter_num, (1, 1), strides=stride)) else: self.downsample = lambda x:x #前向传播 def call(self, inputs, training=None): # [b, h, w, c] out = self.conv1(inputs) out = self.bn1(out) out = self.relu(out) out = self.conv2(out) out = self.bn2(out) identity = self.downsample(inputs) output = layers.add([out, identity]) output = tf.nn.relu(output) return output#建立残差网络模型class ResNet(keras.Model): #通过在__init__中定义层的实现 def __init__(self, layer_dims, num_classes=100): #resnet18的layer_dims为[2, 2, 2, 2] super(ResNet, self).__init__() self.stem = Sequential([layers.Conv2D(64, (3, 3), strides=(1, 1)), layers.BatchNormalization(), layers.Activation('relu'), layers.MaxPool2D(pool_size=(2, 2), strides=(1, 1), padding='same') ]) self.layer1 = self.build_resblock(64, layer_dims[0]) self.layer2 = self.build_resblock(128, layer_dims[1], stride=2) self.layer3 = self.build_resblock(256, layer_dims[2], stride=2) self.layer4 = self.build_resblock(512, layer_dims[3], stride=2) # output: [b, 512, h, w], #GlobalAveragePooling2D(是平均池化的一个特例,主要是用来解决全连接的问题)是将输入特征图的每一个通道求平均得到一个数值,它不需要指定pool_size和strides等参数。返回的tensor是[batch_size, channels],例如:128个9*9的feature map,对每个feature map取最大值直接得到一个128维的特征向量。 self.avgpool = layers.GlobalAveragePooling2D() self.fc = layers.Dense(num_classes) #在call函数中实现前向过程 def call(self, inputs, training=None): x = self.stem(inputs) x = self.layer1(x) x = self.layer2(x) x = self.layer3(x) x = self.layer4(x) # [b, c] x = self.avgpool(x) # [b, 100] x = self.fc(x) return x #串联多个残差块 def build_resblock(self, filter_num, blocks, stride=1): res_blocks = Sequential() # may down sample res_blocks.add(BasicBlock(filter_num, stride)) for _ in range(1, blocks): res_blocks.add(BasicBlock(filter_num, stride=1)) return res_blocksdef resnet18(): return ResNet([2, 2, 2, 2])
总结
今天介绍了图像分类中各种CNN的迭代过程,网络越来越深,网络的复杂度也不断增加,但图像分类的准确度连年上升。下一节开始介绍另一大类研究方向–图像识别,包括各种各样新的网络结构和算法,敬请期待?